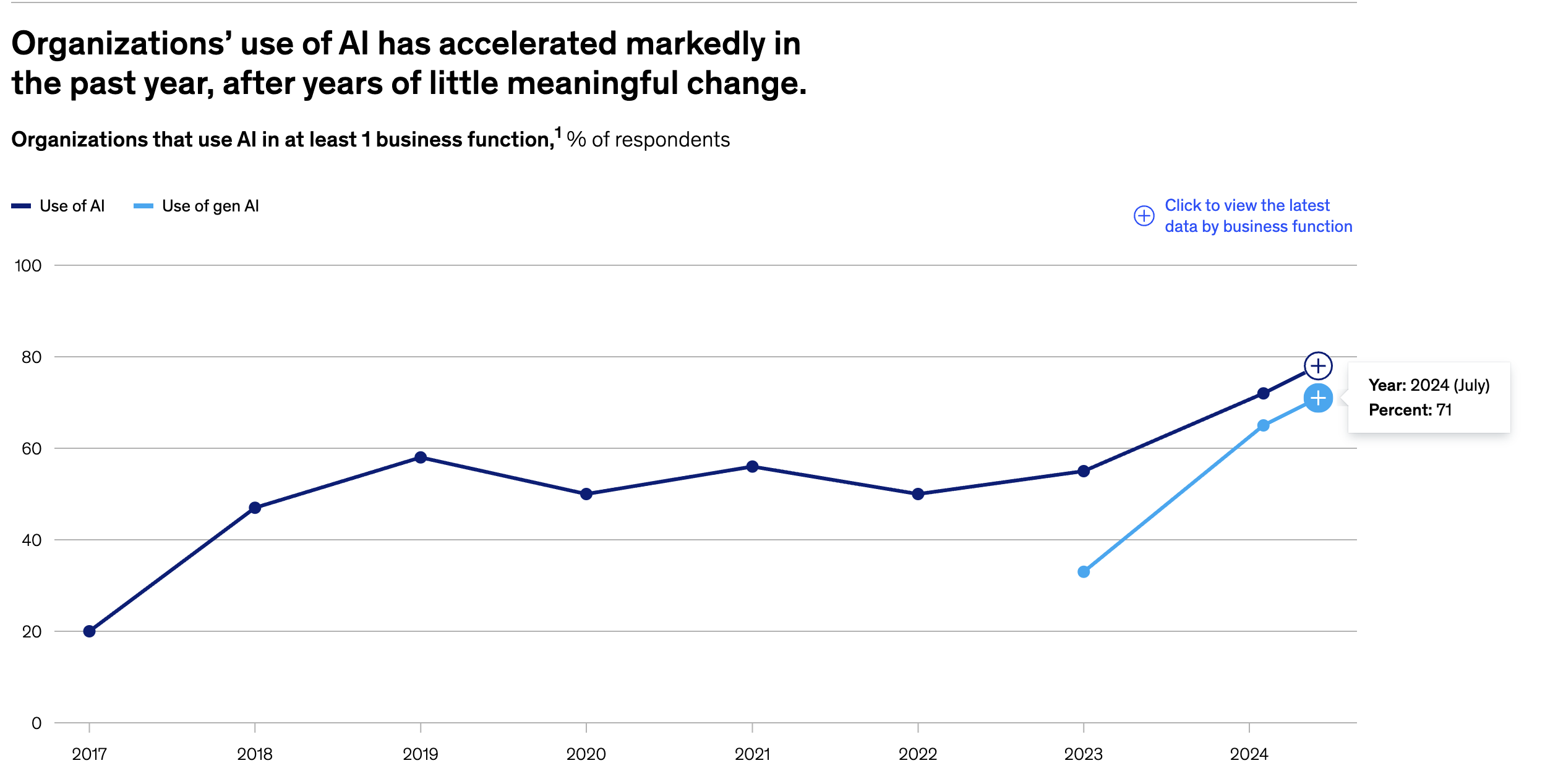
The adoption of generative AI by organizations has more than doubled in the past two years, from 33% in 2023 to 71% in 2024.
Yet McKinsey's latest global survey reveals that only 1% of company executives describe their generative AI rollouts as "mature."
This stark contrast highlights a critical reality: while enterprises are rapidly embracing AI agents, the best practices and playbooks for adopting agentic workflows remain largely uncharted territory.
We're witnessing the dawn of the Agentic Era—a fundamental shift where AI systems evolve from passive tools that respond to prompts into autonomous agents that perceive environments, make decisions, and take actions with minimal human intervention.
Every enterprise is now wrestling with the same question: how do we harness these autonomous workflows to create actual business value without undermining our core products or watching our balance sheets implode?
To get some real-world perspective on this challenge, I recently sat down with Amar Doshi, SVP of Product at 6Sense. Amar isn't just another tech executive theorizing about AI—he's been in the trenches, building products that evolved from basic analytics to sophisticated AI systems that predict customer behavior and automate complex workflows.
.jpg)
This article combines their practical conversation with McKinsey's latest research to offer a realistic roadmap for navigating enterprise agent adoption—based on what's actually working in the field today.
Understanding AI Agents in the Enterprise Context
To grasp what makes AI agents transformative for enterprises, we need to understand how they differ from traditional software applications.
"Agents are overhyped. Every next person I meet is trying to call every little feature they have an agent, right? I feel like we need to come up with a better definition of what an agent is."
- Amar Doshi candidly observes.
True agents, according to Doshi, demonstrate a sophisticated combination of capabilities—perceiving environments, making decisions, and taking complex, multi-step actions autonomously.
They represent the evolution of AI from descriptive (explaining what's happening) to predictive (forecasting what might happen) to prescriptive (recommending what to do) and finally to generative and agentic (actually doing it).
But honestly, defining what ‘Agent’ means is easier said than done. This latest article by TechCrunch is a good place to start.
If you're really curious about diving deeper into what constitutes a true AI agent, check our own exploration in ‘The Anatomy of AI Agents: Understanding the Building Blocks of Autonomous AI.’
When software starts making decisions and taking actions with increasing autonomy, we need new rules of engagement. And that’s exactly why understanding the new Agentic playbook is crucial for Enterprises small and large.
Navigating the New Rules of the Agentic Era
We're entering uncharted territory here. The Agentic Era demands new rules, new guidelines, and new ways of thinking about enterprise software. The old playbooks won't cut it anymore.
Based on Amar Doshi's insights and McKinsey's research, here are the key considerations enterprises must address when adopting agentic workflows:
- Security & Compliance: Non-negotiable foundations for enterprise adoption
- Outcomes Over Black Boxes: Focusing on results, not technical details
- The One-Way Door Problem: Making integration decisions that won't box you in
- Data Quality & Contextual Relevance: Ensuring your agents are well-informed
- The Human Element: Building the right teams and processes
- Strategic Timing: Finding the balance between leading and lagging
- The Co-pilot Approach: Positioning agents as enhancers, not replacements
Let's dive into each of these critical areas.
The Non-negotiables: Security and Compliance

When it comes to enterprise agent adoption, security and compliance aren't optional considerations—they're foundational requirements.
‘Security and compliance are very important... Our customers demand that from us. We have DPAs, sub-processor agreements... making sure we handle data the right way and our vendors handle data the right way. Those are things you can't compromise on.’ - Amar Doshi on the Adopted Podcast.
This isn't just one product leader's opinion. McKinsey's data shows that risk and compliance (57%) and data governance (46%) are the two most frequently centralized elements of AI deployment. Companies aren't leaving these to chance or treating them as afterthoughts.
Let me paint a scenario: Imagine you're a healthcare company implementing an AI agent to help doctors analyze patient data. One day, your agent accidentally exposes protected health information because a vendor's security protocols weren't properly vetted. The damage to patient trust, regulatory penalties, and your reputation would be devastating.
For enterprises evaluating AI agents, this translates to several practical considerations:
- Ironclad data governance frameworks that clearly define what data agents can access and how they use it
- Clear DPAs and sub-processor agreements with vendors that spell out data handling responsibilities
- Consistent monitoring for all AI outputs with appropriate human oversight
Speaking of oversight, practices vary dramatically. McKinsey found that 27% of companies review all AI-generated content before use, while a similar percentage checks less than 20%. This trend is clearly going to change, with the percentage of companies reviewing AI generated content only going up.
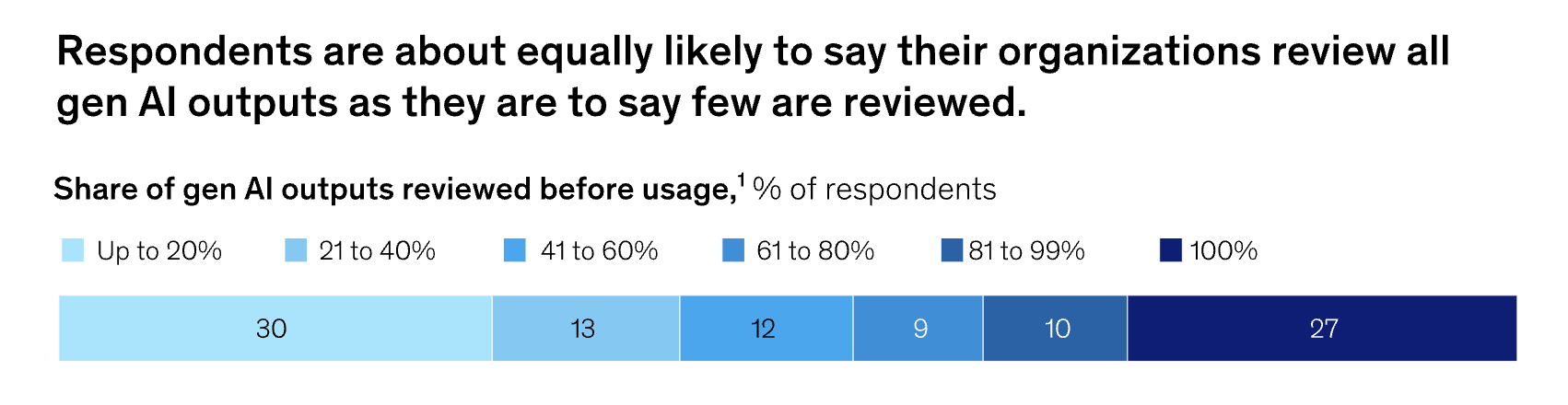
Outcomes-First Evaluation: Beyond Black Box Models
When evaluating AI agents, enterprises often get caught up in the technical details of how models work. Amar Doshi offers a more pragmatic perspective.
"What's the detail going to help you with? At the end, you should be concerned with outcomes," he observes. "A lot of folks have this intellectual curiosity... they need to know exactly what the model is doing... but the product doesn't really need to completely unravel the black box."
I've seen this firsthand in enterprise software evaluations. Technical leaders can spend weeks debating the merits of different embedding techniques or attention mechanisms, while business stakeholders just want to know: "Will this make our customer service reps more efficient?"
This outcomes-focused approach aligns with McKinsey's findings on value creation. The survey revealed that tracking well-defined KPIs for generative AI solutions is the practice most correlated with bottom-line impact.
Yet despite this importance, less than 20% of organizations currently track such metrics.
What does an outcomes-focused approach look like in practice?
- Match agent evaluations to specific business objectives that matter to your organization
- Establish clear, measurable KPIs that tell you whether the agent is delivering value
- Run targeted pilots with concrete success metrics before full-scale deployment
The McKinsey data demonstrates that this approach is yielding results. In the second half of 2024, 70% of organizations using gen AI in strategy and corporate finance reported revenue increases, up from 43% in early 2024.
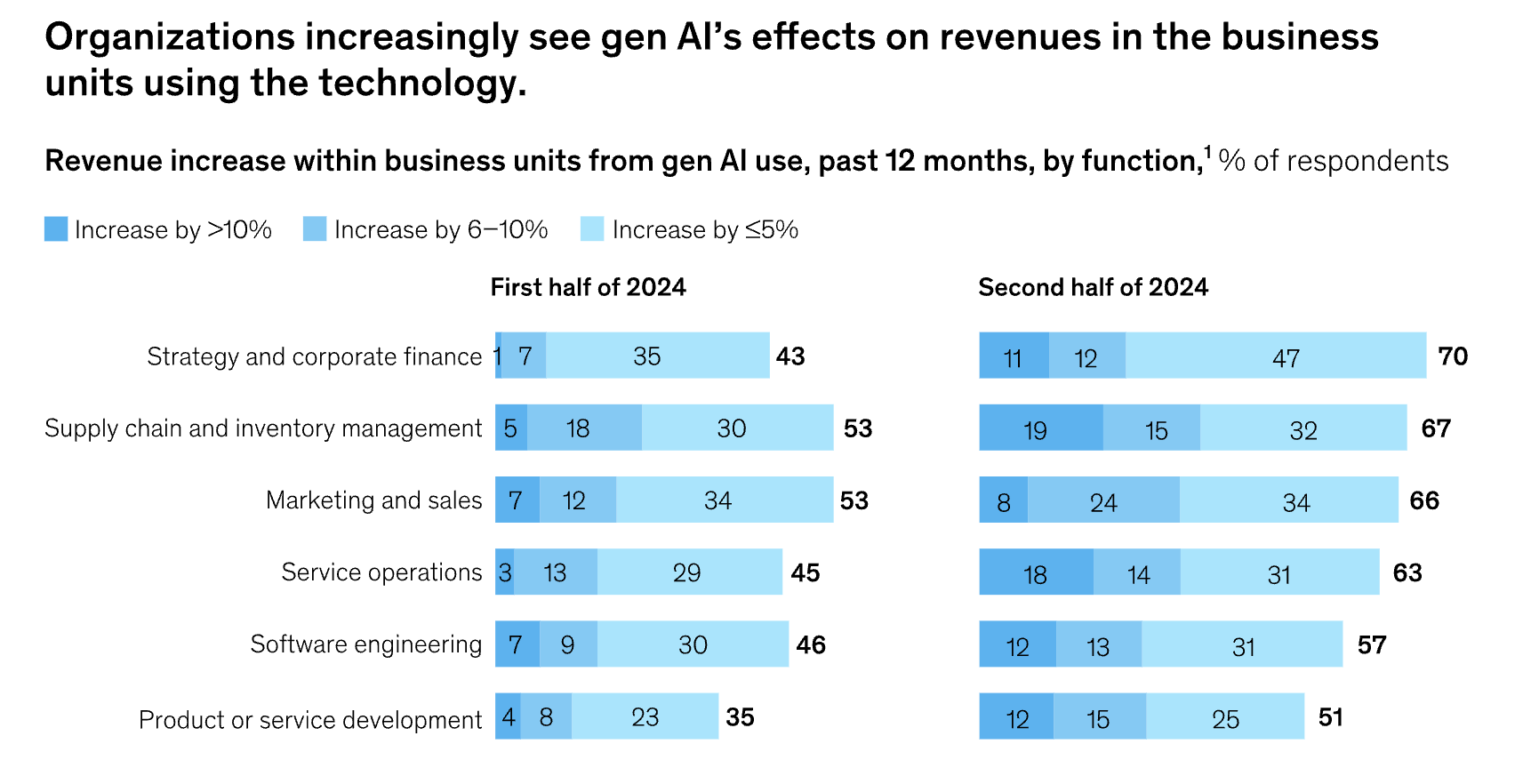
The "One-Way Door" Problem in Enterprise Tech
Enterprise technology decisions—particularly those involving AI agents—represent what Amar Doshi calls "one-way doors," borrowing from Jeff Bezos' decision framework.
"Some products, once integrated, are difficult to replace. Enterprises have responsibility to evaluate well. In product, we don't buy any tech without an evaluation... once they make it into your product pipeline, serving the needs of your end users, it's very difficult to replace them." - Amar Doshi on the Adopted Podcast.
Think about it this way: You're building a house, and you decide to install a particular type of electrical system. Six months later, you realize it doesn't meet your needs, but replacing it would require tearing down walls, redoing the flooring, and essentially rebuilding significant portions of your home. That's the "one-way door" problem with enterprise AI integration.
For enterprises evaluating agent solutions, this means:
- Conducting thorough technical due diligence that looks beyond demos and promises
- Considering long-term integration implications and potential exit strategies
- Evaluating vendor stability and product roadmaps to avoid betting on dead-end technologies
Large organizations (>$500M in revenue) are particularly deliberate in their approach, being twice as likely as smaller companies to establish clear roadmaps for AI adoption with phased rollouts.
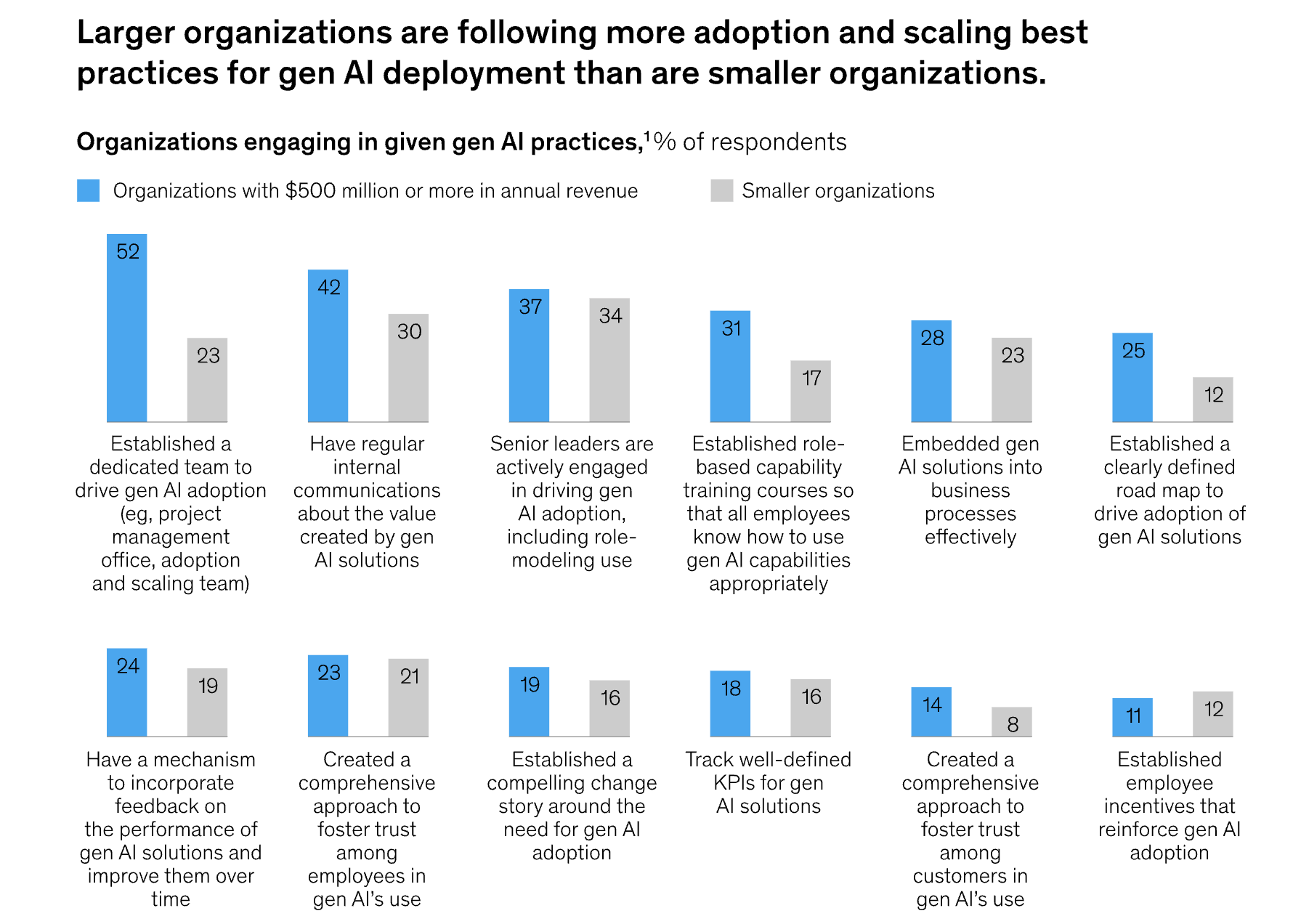
Data Quality and Contextual Relevance
During the podcast, Deepak Anchala emphasized a critical principle that Amar Doshi strongly endorsed:
"AI is as good as the data it's working on—garbage in, garbage out."
This principle is especially significant for enterprise-grade AI agents, which must produce reliable, accurate, and consistent outputs. The McKinsey survey confirms this priority: inaccuracy is the top risk that organizations are actively mitigating (48% of respondents), reflecting awareness of this fundamental challenge.
Here's a real-world example I've encountered: A sales operations team implemented an AI agent to forecast pipeline based on historical data. The forecasts were consistently off-target, causing the team to miss revenue projections.
The culprit? The historical data included several accounts with unusual deal structures that skewed the model's understanding of typical sales cycles.
The agent wasn't "wrong"—it was working with incomplete context.
Enterprises must focus on:
- High-quality, well-structured data inputs that accurately represent the business reality
- Domain-specific contextual knowledge that helps agents understand the nuances of your industry
- Continuous validation mechanisms that catch drift between what the agent "knows" and what's true
What we're witnessing here is a fundamental paradigm shift in how enterprises need to prepare for an agentic world. We're moving beyond traditional UX (user experience) to what we call AX—Agentic Experience.
This new approach requires organizations to think deeply about how humans and AI agents collaborate, how feedback loops operate, and how data flows through these new systems.
We explored this concept in depth in our recent blog on ‘The Rise of Agent Experience: Designing for AI as the Third User’.
The Human Element: People, Process, and Technology
There's a persistent myth that AI implementation is primarily a technical challenge. Install the right software, connect the right APIs, and you're set. The reality couldn't be more different.
Amar Doshi emphasizes this human dimension -
‘It's people, process, and technology. People have a big role to play... customer success, strategic services, adoption consulting teams. You need a tribe to help customers do more with your product.’
The McKinsey survey supports this people-first perspective, revealing that organizations are both hiring for AI-specific roles (13% have hired AI compliance specialists) and re-skilling existing employees.
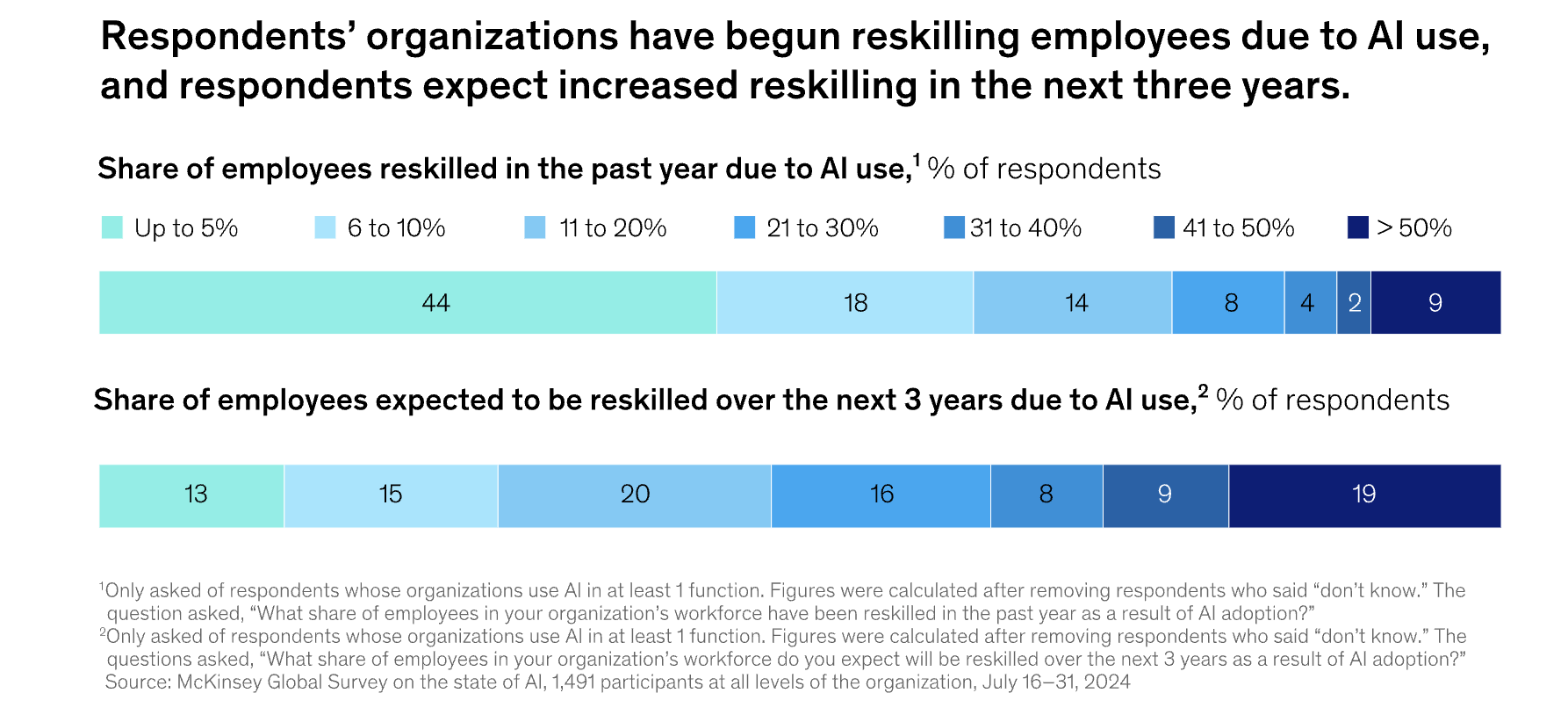
A full 44% of companies have re-skilled up to 10% of their workforce due to AI adoption in the past year, with 19% expecting to re-skill more than 50% of employees over the next three years.
Successful enterprises are:
- Building dedicated adoption and scaling teams that bridge the gap between technology and users
- Creating role-based capability training that helps people understand how agents can enhance their specific jobs
- Establishing feedback loops where human input continuously improves agent performance
Larger organizations ($500M+ revenue) are leading in these areas, being more than twice as likely as smaller companies to have established dedicated teams driving AI adoption.
Strategic Considerations: Leading or Lagging?
The million-dollar question for many enterprises: should we jump in now or wait until things are more mature?
Amar Doshi offers this perspective:
‘You have to make some bets and say, the cost of being late to this game is that you'll have a later start. You probably will face competition if your competitors and new entrants go down that path.’
This should remind us of the early days of cloud computing. Some companies jumped in immediately, experiencing both the benefits of early adoption and the growing pains of immature technology. Others waited until the technology stabilized, avoiding early pitfalls but finding themselves years behind in capabilities and organizational learning. There's no perfect answer—just strategic tradeoffs.
McKinsey's findings reveal that early adopters are already seeing results. Among organizations using generative AI, cost reductions are being reported across multiple functions, with human resources (50%) and service operations (45%) leading the way.
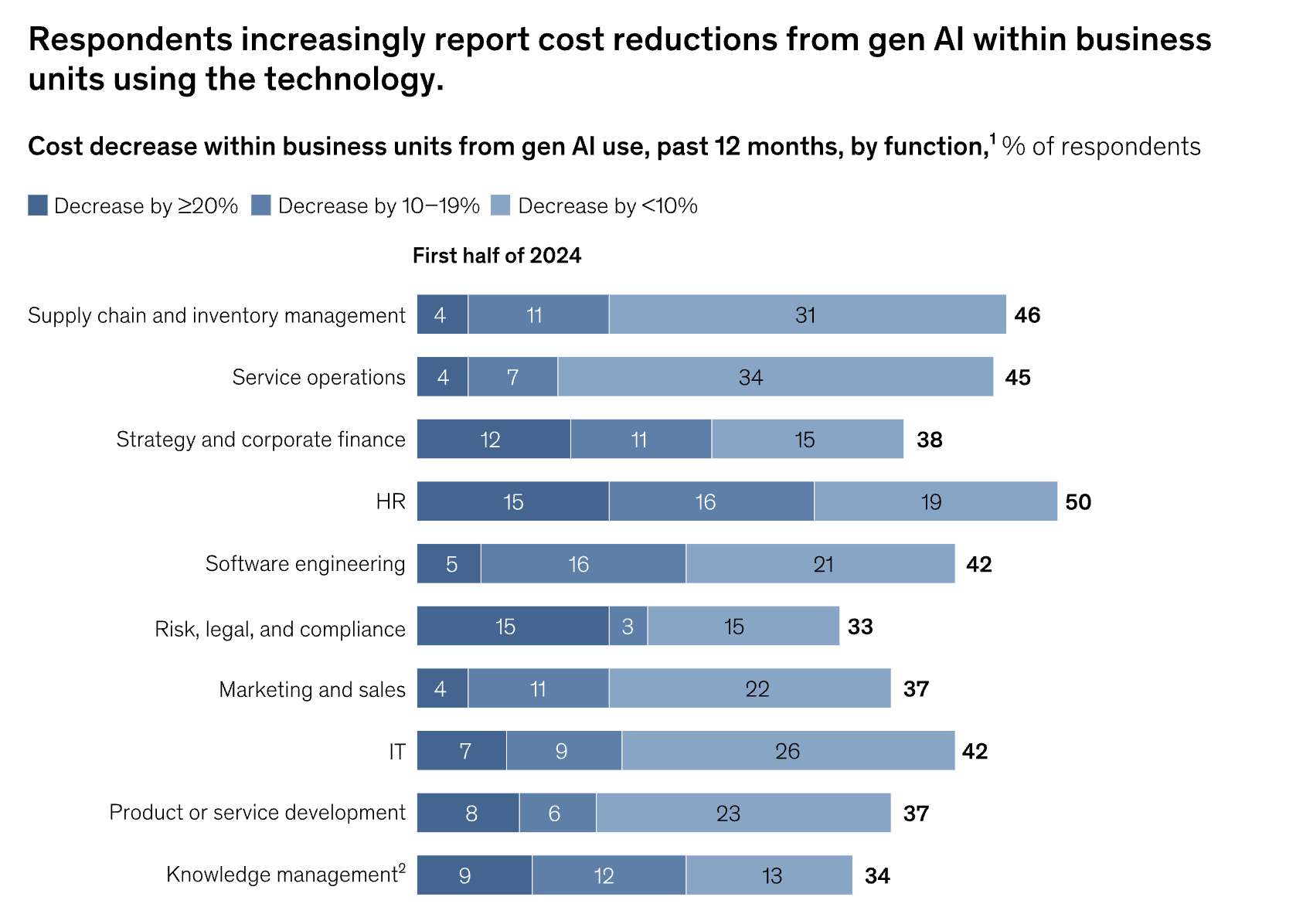
For enterprises, this suggests a measured approach:
- Start with high-impact, low-risk use cases that can deliver visible wins
- Develop phased implementation plans that build capability incrementally
- Invest in learning and experimentation that will pay dividends as the technology matures
The Future: AI Agents as Co-pilots, Not Replacements
So where is all this headed? Are agents going to replace our software, our jobs, our coworkers?
Amar Doshi offers a refreshingly realistic perspective:
"AI agents are almost like co-pilots to SaaS software. They make SaaS better. I don't think it takes away traditional software as a service. People still may want to consume data and do things in different ways."
We’re big fans of this co-pilot metaphor. Think about actual airplane co-pilots—they don't replace the captain; they enhance the captain's capabilities, handle routine tasks, provide backup, and sometimes spot things the captain might miss.
That's exactly how we should think about AI agents in the enterprise.
Conclusion: The Strategic Imperative
We're in the opening chapter of the Agentic Era. The technology is powerful but immature. The opportunities are enormous but so are the challenges. And the enterprises that will thrive aren't necessarily the ones with the most advanced AI—they're the ones that implement AI most thoughtfully.
The path forward isn't about blindly embracing every new AI agent capability, nor is it about waiting on the sidelines as competitors forge ahead. Instead, it's about making strategic bets, implementing thoughtful governance, and redesigning workflows to capture genuine value.
As Amar Doshi wisely observes,
"At some point, when AI learns applications, humans won't need to."
The enterprises that thrive will be those that begin preparing for that future today—balancing innovation with responsibility, technology with humanity, and experimentation with strategic focus.