Throughout technology history, new innovations have consistently birthed new business models.
The internet gave us software-as-a-service. Mobile created the app economy.
And now, we're witnessing another seismic shift as autonomous AI agents - systems that can perform complex tasks with minimal human supervision - fundamentally change how software is valued and priced.
Think about it - when software stops being just a tool and starts functioning more like a teammate, everything about how we measure its worth needs to change.
In this piece, we explore this pricing revolution happening right before our eyes – examining how we got here, the experiments currently underway, and where all this likely leads.
The companies that navigate this transition successfully will likely define the next decade of software economics.
How We Got Here: The Evolution of Software Pricing
To understand today's changes, we need to appreciate the journey of software pricing through three distinct eras:
The Ownership Era (1980s-2000s)
Remember the days of installing software from physical disks?
The original model centered entirely on ownership – companies purchased perpetual licenses with massive upfront payments, paid annual maintenance fees, and that software became a fixed asset. Oracle, SAP, and Microsoft built empires on this approach.

This was firmly in capital expenditure territory. CFOs wrote big checks, depreciated the software over years, and expected it to last. IT departments physically installed patches, and upgrades were major events requiring significant planning.
The Access Era (2000s-2015)
Then came the SaaS revolution, famously symbolized by Salesforce's ‘No Software’ logo – a radical concept at the time. Companies weren't buying software anymore; they were renting access to it.
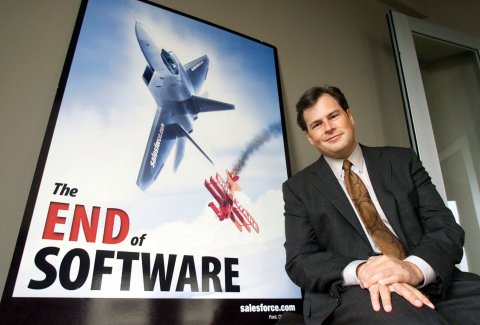
This transformed the entire software consumption model. Massive upfront costs? Gone. Multi-year depreciation schedules? Gone.
Instead, businesses paid predictable monthly fees, typically based on how many employees used the system.
Per-seat pricing dominated this era because it made intuitive sense: more users meant more value received. It scaled naturally with company size and was easy to understand and budget for.
Most importantly, it matched how humans interact with software – each person needs their own login, so each person represents a cost unit.
The Consumption Era (2015-Present)
Around 2015, a shift toward usage-based pricing began taking hold. Twilio charged per message sent. Snowflake charged based on compute and storage consumed. Stripe took a percentage of transactions processed.
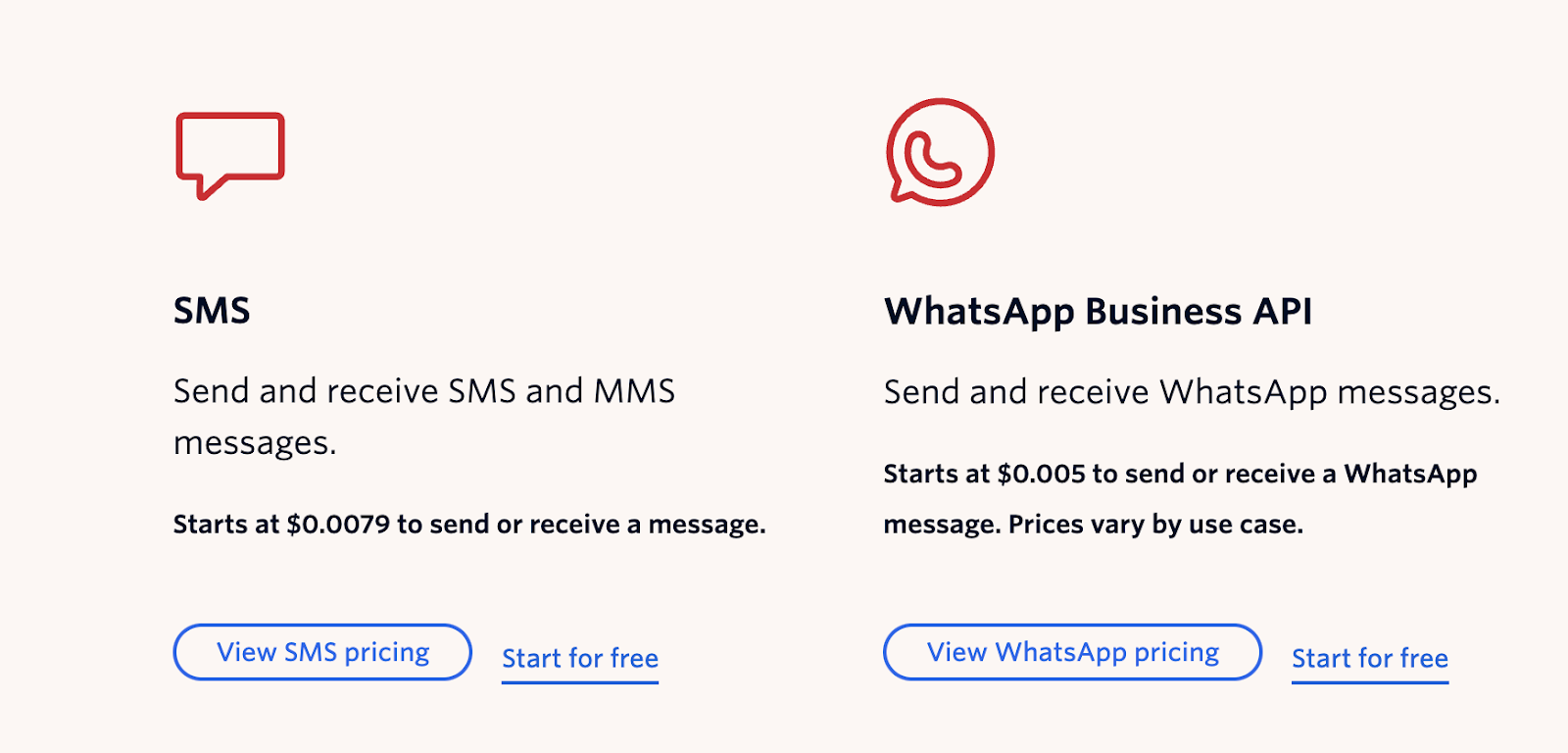
This model recognized that value derived from software wasn't just about access – it was about outcomes. Companies started paying for what they actually used rather than how many employees logged in. For many businesses, this created a tighter alignment between cost and value.
But now we're entering a fourth era, and it's poised to make all these previous transitions look like mere warm-ups.
Why Everything Is Changing
Imagine a customer service leader who just automated away about 40% of their team's workload with a new AI agent system.
It's a massive win for efficiency – until they get the next software bill and discover it isn't any lower despite needing fewer seats.
"Why are we paying the same amount when the software is doing the work now instead of my team?" they might reasonably ask.
That's the million-dollar question at the heart of this transformation.
When we examine why traditional pricing models break down in this new world, three fundamental issues emerge:
The Value-Creation Disconnect
In the world of human-operated software, per-seat pricing worked because each human represented capacity and value creation. But AI agents create value independently of human operators.
Consider a customer service AI that can handle thousands of conversations simultaneously – work that would require dozens of human agents.
Should pricing still be tied to how many humans are supervising the system? The connection between seats and value is rapidly disintegrating.
The Economics of Intelligence
Unlike traditional software, which costs essentially the same to operate whether one customer or a thousand uses it, AI has real, variable costs that scale with usage.
Every interaction with an AI agent consumes computing resources. Companies using third-party AI APIs like GPT-4 pay per token for each interaction. This creates a direct relationship between usage and cost that simply doesn't exist in traditional software.
For software providers, this means AI infrastructure costs can spike unpredictably with usage, creating potential margin crises if pricing doesn't account for these variable costs.
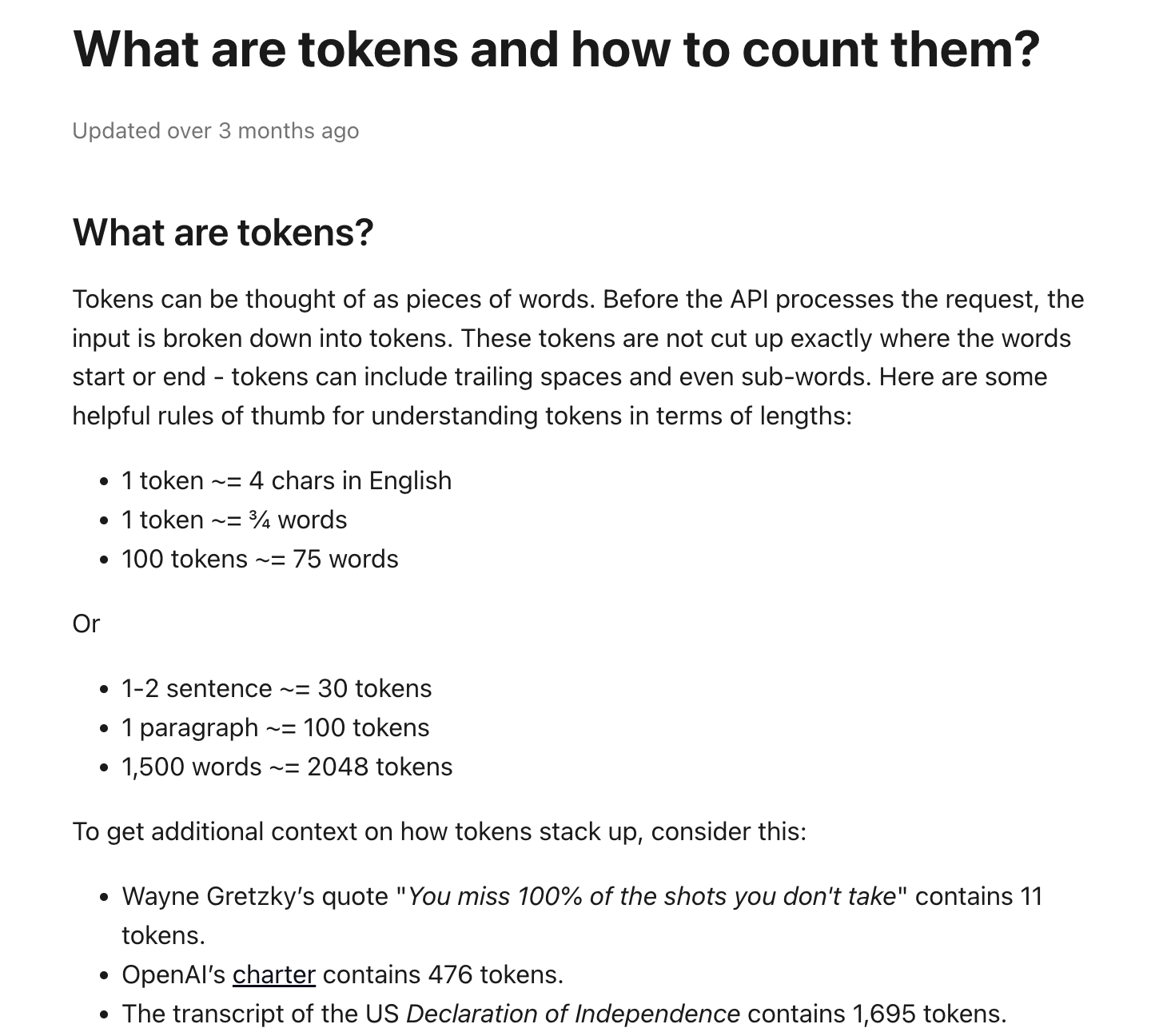
The Efficiency Paradox
Here's perhaps the most perverse aspect: under traditional per-seat models, software vendors actually lose revenue when their AI makes customers more efficient.
If an AI solution reduces a customer's required support headcount by 50%, the vendor's revenue drops by 50% under a per-agent pricing model – despite delivering MORE value. No business wants to innovate itself into lower revenues, yet that's exactly what happens with conventional pricing in the AI age.
The New Playbook: Pricing Models for the AI Age
So what solutions are emerging as companies navigate this transition? Let's look at four innovative approaches taking shape.
Agent Capacity Models
Think of this as hiring digital employees with a monthly salary. Instead of paying per human seat, you pay a subscription for each AI agent deployed.
11x.ai offers "Alice," an AI Sales Development Representative at a fixed monthly cost. Each "Alice" handles outreach emails and research like a human SDR but at a fraction of the cost.
Devin.ai sells AI software engineers on subscription, costing roughly what a junior developer's monthly salary might be.
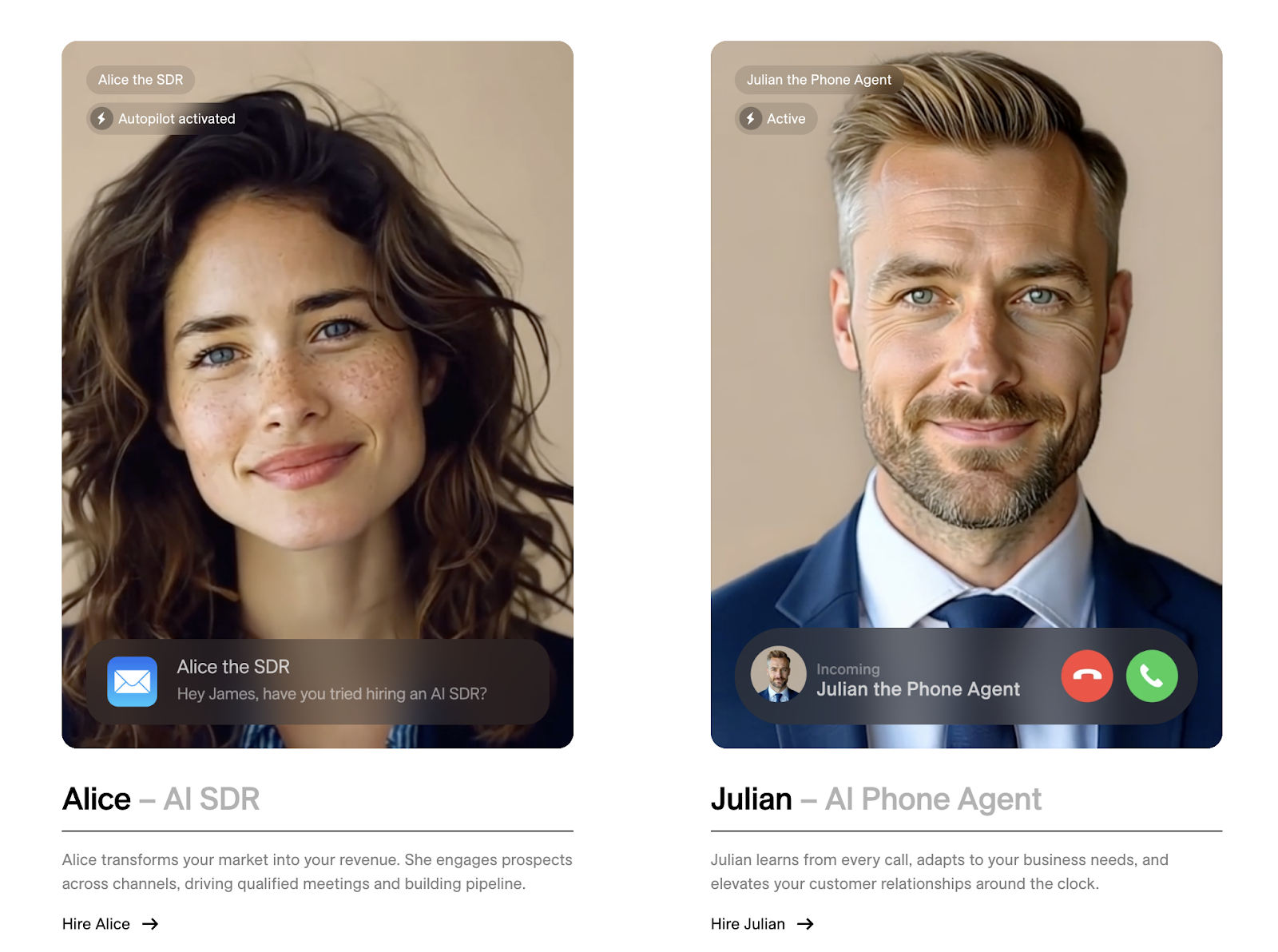
Outcome-Based Pricing
The purest form of value alignment – you only pay when the AI successfully delivers results. No success? No charge.
Intercom charges ~$0.99 per successfully resolved support ticket with its Fin AI agent. If the AI can't resolve the issue and hands off to a human, you don't pay a cent.

Value-Share Models
These tie pricing directly to financial impact, with vendors taking a percentage of the measurable cost savings or revenue generated.
Chargeflow keeps ~25% of the value of chargebacks it successfully reverses for e-commerce companies. The vendor only wins when the customer sees tangible benefits.

Hybrid Approaches
Combining predictable base subscriptions with variable components tied to usage or outcomes – offering the best of both worlds.
Salesforce charges $2 per conversation for its Agentforce platform on top of base platform fees.
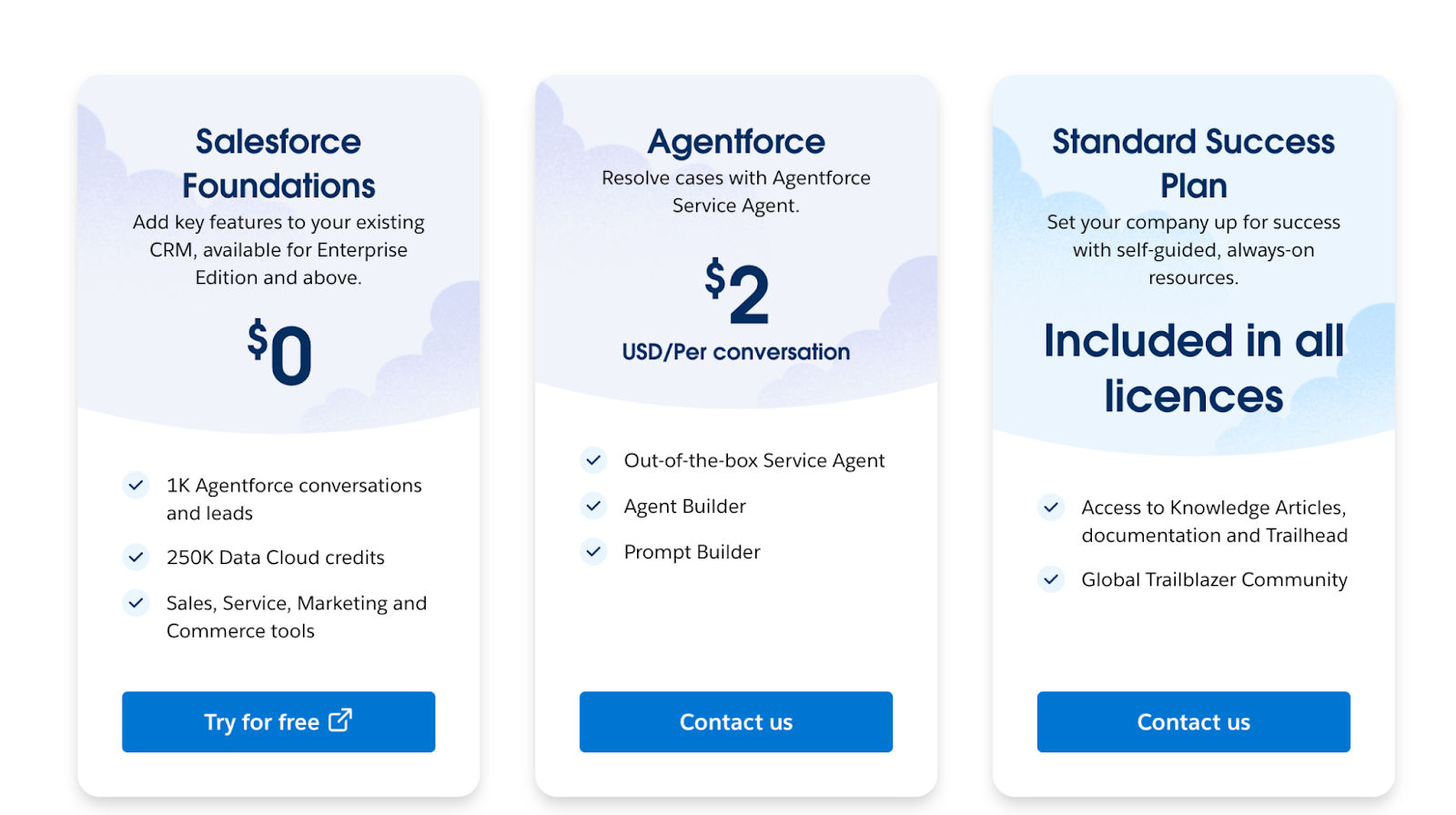
Challenges With New Pricing Models
While these innovative approaches better align with AI's value creation, they introduce significant challenges that both vendors and customers are struggling to navigate:
Budget Unpredictability
Finance teams are built around predictable expenses. When costs vary based on usage or outcomes, budgeting becomes considerably more complex.
In this podcast, Eoghan McCabe, Intercom's CEO, acknowledges this challenge directly: "Part of the problem is the price is not predictable. If I buy a hundred seats, I know how much I'm going to pay you. But if you're just going to charge me for how much your bot decides to do work, how much am I paying?"
This uncertainty can create friction in the purchasing process, as finance teams struggle to forecast and approve variable costs.
Operational Complexity
For vendors, these new models require sophisticated metering, analytics, and billing infrastructure. Companies must track granular usage data, apply complex pricing rules, and provide transparent reporting to customers – all while maintaining performance.
Building these capabilities requires significant investment and expertise.
Many organizations find their existing billing systems simply can't handle the complexity of these new models, requiring additional technology investments or partnerships.
The Education Gap
Both sales teams and customers need to understand these new models and their implications. Sales conversations become more consultative, focusing on value rather than features, and require more sophisticated ROI justifications. This transformation doesn't happen overnight.
Core Principles for Designing AI-Native Pricing
As we navigate this transition, several principles can guide successful pricing strategies for AI-powered software:
1. Know Your Customer Segments
Different customers have different priorities.
Enterprise customers often prioritize predictability and governance over per-unit pricing efficiency, while SMBs might prefer low upfront costs with pay-as-you-grow models.
Design your pricing strategy around your specific audience's buying process and financial priorities.
2. Prioritize Simplicity
Despite the temptation to capture every dimension of value in your pricing, simplicity nearly always wins in the market. Customers need to easily understand what they're paying for and forecast costs reliably.
If your pricing requires a spreadsheet to calculate, it's probably too complex.
3. Connect Price Directly to Value
Establish a clear connection between what customers pay and the value they receive. For outcome-based models, precisely define what constitutes "success."
For usage models, ensure the metrics you charge for genuinely reflect value creation from the customer's perspective.
4. Understand Your Unit Economics
Maintain a solid grasp of your underlying costs, particularly for AI operations. While pricing shouldn't be solely cost-based, knowing your unit economics helps set sustainable prices and defend margins.
This is especially crucial given the variable costs of AI inference.
5. Build in Guardrails
Even with usage-based elements, provide mechanisms like spending caps, volume discounts, or usage alerts to make costs more predictable and prevent billing surprises. These guardrails build trust and reduce adoption friction.
The Road Ahead: Future Trends in AI Pricing
Let's be honest – making predictions about AI pricing feels almost foolish given how rapidly this space is evolving.
What seems cutting-edge today might be obsolete in six months. The only certainty is uncertainty.
But hey, we're going to try anyway! Here's our best shot at where things might be heading:
- Standardization of Metrics – Common units like ‘agent-hours’ or ‘task completions’ will emerge, making comparison shopping possible across vendors (similar to how cloud computing standardized on instance-hours).
- Tiered Outcome Pricing – Simple tasks will have base pricing while complex outcomes command premiums. In fact, recent news says Open AI might be considering charging $2000 premiums for a PHD Level Model.
- Performance Guarantees – Vendors will differentiate with SLAs offering money-back guarantees for accuracy, speed, or completion rates – shifting risk from customers to providers.
- Industry Vertical Specialization – Pricing will fragment by domain: legal AI charging per contract, healthcare AI per diagnosis assisted, financial AI per risk assessment – each with metrics that match their unique value drivers.
In this new era companies that cling to legacy pricing models while delivering AI-native capabilities will increasingly find themselves in an untenable position, unable to capture the true value they create.
The companies that will thrive won't just have the most capable AI – they'll have pricing models that accurately reflect and capture the value their technology delivers.
Those who master this transition will not only ensure sustainable growth but will help define the economic relationships of our AI-powered future.