The battle lines are being drawn.
OpenAI recently released their Agents SDK. Microsoft continues expanding Copilot across their ecosystem. Google is enhancing Gemini's capabilities. Anthropic is championing open protocols.
Everyone wants their approach to become the standard for how AI agents operate.
It feels like we're watching the browser wars or mobile OS battles all over again, except this time, the stakes are even higher. Because whoever controls how AI agents operate - could shape the next decade of computing.
But the core narrative remains the same - is the future open source or close walled?
The question isn't just technical—it's also part philosophical.
Will our AI agents operate in open, interoperable ecosystems where they can freely communicate across platforms? Or will they be confined to walled gardens controlled by whoever built them?
There’s a lot happening and we wrote this piece to take heed of the situation and make some sense of what is to come.
The AI Agent Spectrum: Open vs. Closed Ecosystems
Picture two restaurants. One has a secret recipe they guard zealously—you can enjoy their amazing dish, but only by dining with them.
The other publishes their recipe openly—you can make it at home, modify it to your taste, or even serve it in your own restaurant with improvements.That's essentially the difference between closed and open AI ecosystems.
An open AI ecosystem involves models and tools whose code or weights are publicly available under permissive licenses. Developers can download, modify, and build upon these foundations to create custom solutions. Meta's LLaMA family and Mistral's open models exemplify this approach.
“Open source will ensure that more people around the world have access to the benefits and opportunities of A.I., that power isn’t concentrated in the hands of a small number of companies, and that the technology can be deployed more evenly and safely across society" - Mr. Mark Zuckerberg.
A closed ecosystem, by contrast, keeps the core technology private. You can use the AI through controlled APIs or interfaces, but you can't see how it works or modify it to suit specific needs. OpenAI's GPT-4 and Google's Gemini operate this way—powerful but proprietary.
We've seen similar patterns throughout tech history. AOL once tried to keep everyone within its walled garden before the open web won out. BlackBerry's proprietary messaging eventually gave way to standard protocols. Even operating systems like Windows have become more open over time in response to market demands.
With AI agents potentially becoming our primary digital interfaces, the approach that prevails will determine whether our AI future is controlled by a few companies or shaped by many contributors.
The Current Landscape: Major Players and Their Strategies
As the agent ecosystem takes shape, each major player is pursuing a distinct strategy that reveals their vision for AI's future:
OpenAI has adopted a somewhat contradictory approach. Their models remain firmly closed, accessible only through their API. Their new Agents SDK offers some openness—it's technically open source and can work with non-OpenAI models. However, as industry observers have noted, their APIs are designed in ways that make switching providers difficult—creating subtle but effective lock-in.
Microsoft has built perhaps the most comprehensive enterprise agent ecosystem through Copilot, Azure AI, and Windows integration. Their approach is hybrid—their platform is proprietary, but they support multiple models, including open-source ones. Microsoft wants to be the enterprise AI platform regardless of which models you prefer, positioning themselves as the Switzerland of enterprise AI.
Anthropic has positioned Claude as a premium model while championing open standards. They've spearheaded the Model Context Protocol (MCP), creating a standardized way for AI models to connect with external tools and data. Their strategy balances commercial interests with ecosystem development.
Google/DeepMind started firmly closed but is showing signs of openness. After initially keeping Gemini behind Google's walls, they released Gemma 3 as an open-source model. They seem caught between protecting their investments and engaging with the innovation happening in open communities.
Meta has emerged as the open-source disruptor by releasing powerful models like LLaMA 2 freely. Their approach aims to democratize access to powerful AI while ensuring no single vendor dominates the landscape.
As Mark Zuckerberg explained, "Open source drives innovation because it enables many more developers to build with new technology."
Emerging players like Mistral AI and Stability AI are further pushing the open frontier, releasing increasingly capable models under permissive licenses and challenging the idea that powerful AI must be proprietary.
This diversity of approaches creates both opportunities and challenges. For developers and enterprises, it means navigating a complex landscape of options with different tradeoffs. For the industry as a whole, it raises the question of whether these different approaches can find common ground that enables interoperability without sacrificing innovation.
The Business Equation: Open vs. Closed AI Ecosystems
Let's cut to the chase on what enterprises really face when choosing between open and closed AI approaches. I've seen both sides of this equation play out across industries, and the tradeoffs are pretty clear.
Closed Ecosystems: The Ready-Made Solution

Closed AI ecosystems like OpenAI's GPT-4 or Microsoft's Copilot suite offer a turnkey experience. You're essentially buying a premium service that handles the complex AI machinery for you.
The upside? You get polished tools that work right away. Your teams can start generating content, analyzing data, and automating workflows without needing deep AI expertise. For regulated industries, there's comfort in knowing that compliance and security responsibilities largely fall on the provider's shoulders.
But there's always a catch. As you grow dependent on these systems, switching becomes increasingly difficult—classic vendor lock-in. Your AI future is essentially hitched to someone else's roadmap and pricing model. I've watched companies panic when their provider doubled prices or deprecated features they relied on.
Control is another major issue. When you need specialized capabilities that don't align with your provider's priorities, you're simply out of luck. And at scale, those per-token or per-query charges that seemed reasonable at first can quickly balloon into budget-busting expenses.
Open Ecosystems: The DIY Approach
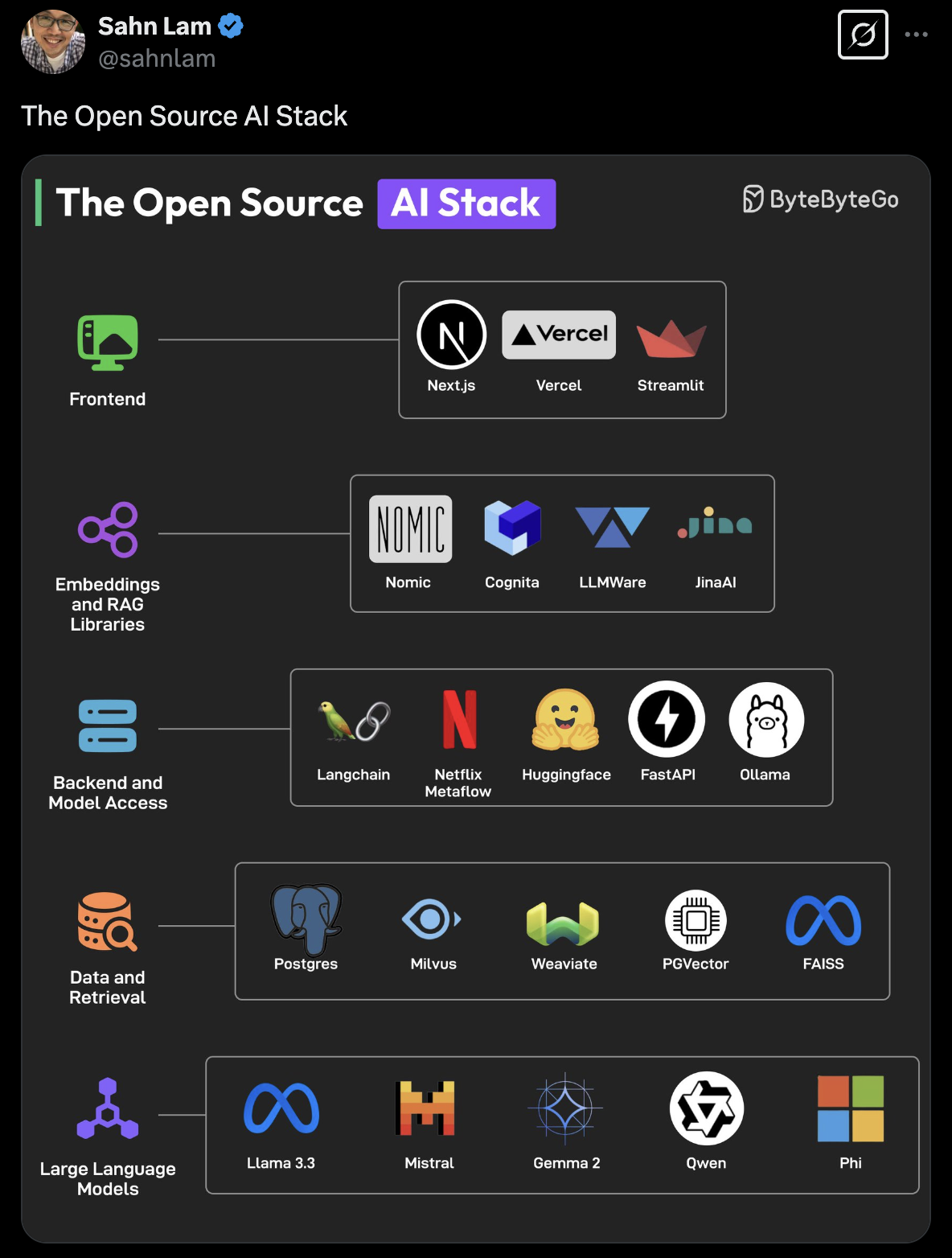
Open AI systems like Meta's LLaMA or Mistral's models flip the script. Rather than paying for a service, you're getting tools you can customize and control.
This approach shines when you need AI tailored to your specific domain. Financial services companies can fine-tune models for their terminology and compliance needs. Healthcare providers can ensure their systems understand medical contexts. This customization often delivers superior results for specialized applications.
The economics also change dramatically at scale. Running your own fine-tuned open models typically costs a fraction of equivalent API calls to proprietary systems—sometimes 5-7× less expensive. That's real money back into your innovation budget.
Perhaps most valuable is the strategic independence. You can deploy these models entirely within your secure infrastructure, keeping sensitive data under your control while still leveraging advanced AI capabilities.
The downside? You'll need technical expertise to make it work. You're now managing AI infrastructure, building safety guardrails, and handling integration challenges that would have been abstracted away in closed systems. It's more work and responsibility—no vendor to blame if something goes wrong.
In reality, most enterprises are finding success with a hybrid approach.
The smart move isn't choosing a side in this debate—it's being strategic about where each approach makes sense for your specific needs.
History's Lessons: Why Openness Usually Wins
But the question still remains - which approach will dominate in the years to come?
The honest answer is that no one knows for certain. But if technology's history is any guide, we have some compelling clues.
Time and again, open ecosystems tend to outgrow and eventually eclipse closed ones. The internet itself triumphed over closed networks like CompuServe and AOL. The open web defeated proprietary information systems. Android's more flexible approach helped it achieve dominant global market share over iOS.
This pattern hasn't gone unnoticed even within the tech giants themselves. In 2023, an internal memo from a Google engineer went viral after being leaked. Titled "We Have No Moat," it delivered a stark warning that neither Google nor OpenAI were positioned to win the AI arms race.
.png)
Instead, the engineer pointed to the open-source community as the "third faction" quietly eating their lunch. The memo detailed how open-source models were closing the quality gap "astonishingly quickly" while being faster, more customizable, and more private than their closed counterparts.
Why does this pattern repeat? Open systems create larger networks and foster innovation beyond what any single company can achieve.
A recent Harvard Business School study titled "The Value of Open Source Software" quantifies this impact, finding that open source software creates a staggering $8.8 trillion in value for the global economy.
Perhaps most telling is their finding that firms would need to spend 3.5 times more on software than they currently do if open source didn't exist—a testament to how foundational open approaches have become to our technology infrastructure.
We're already seeing this pattern play out in the AI agent space with the emergence of the Model Context Protocol (MCP).
This "USB-C for AI" creates a standardized way for any AI agent to connect to external tools and data sources. It's a bridge between different AI systems that could make true interoperability possible.
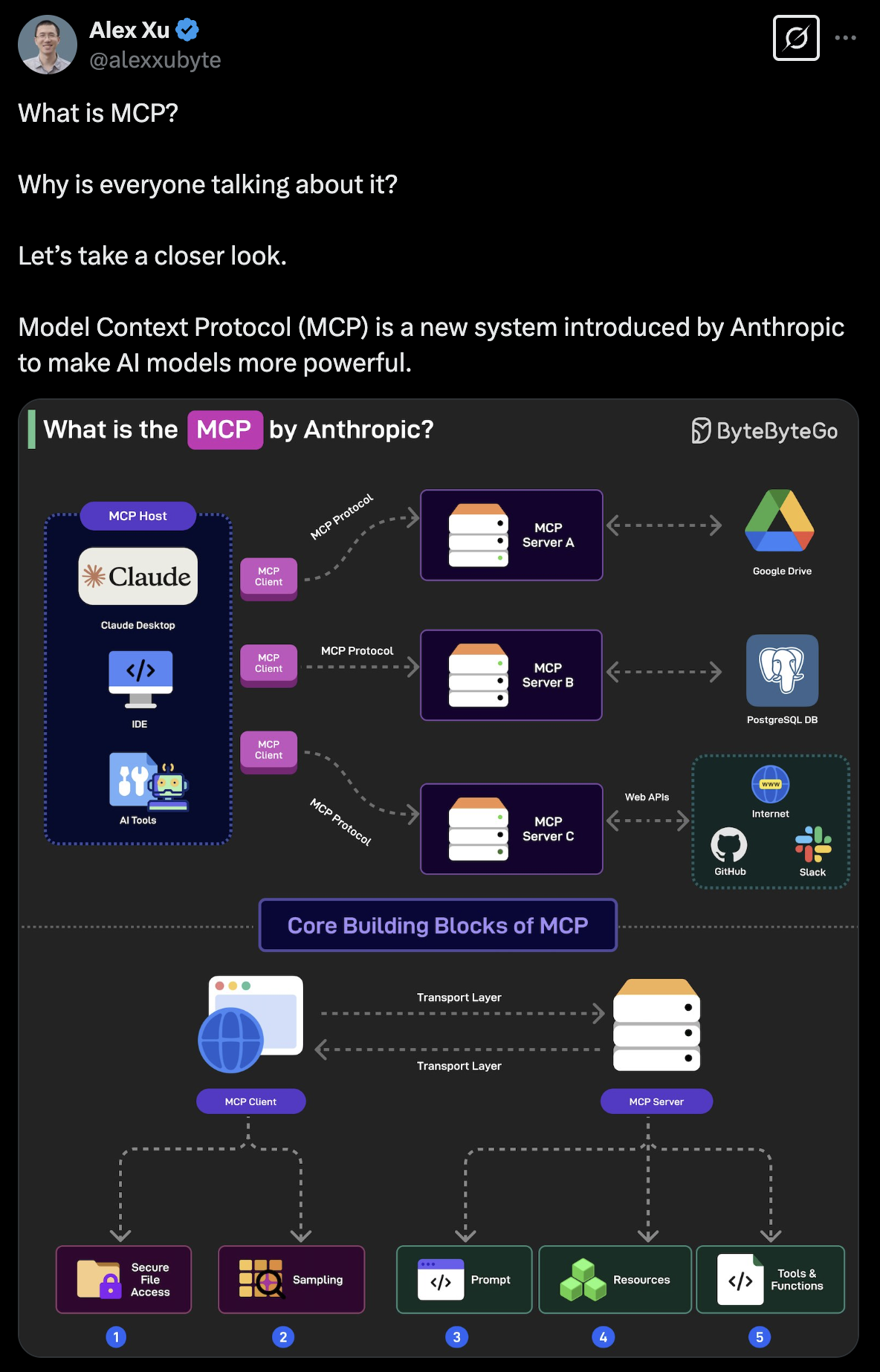
The Case for Interoperability: Building Bridges Between AI Islands
In a conversation on the Adopted Podcast, Matt Biilmann, CEO of Netlify, articulates the power of open agent interoperability perfectly:
"I think building those open standards and opening up the world of our product will give much more power to users and will also get us that much richer ecosystem where you can have your different products and your different agents and there's competition on each of those layers."
This vision of layered competition is compelling. Just as web browsers compete on features while all rendering the same HTML, AI agents could compete on capabilities while all working with the same tools through common interfaces.
The key insight Billmann shares is that agent interoperability creates a win-win scenario. Users gain more choice and flexibility, while the total ecosystem grows larger than any closed system could achieve alone.
"Eventually when it becomes like the standard way of working," Billmann notes, "people will pick products that are better for their agent to work with than tools that are hard for the agent to work with."
Early adopters of MCP, including Anthropic's Claude and various development tools, are showing how this standardized interface can create a more fluid experience. Rather than each application having its own proprietary AI, or each AI being limited to its own ecosystem, MCP enables a "mix and match" approach that benefits everyone.
The Path Forward: Toward a Collaborative AI Future
As we navigate this pivotal moment in AI's evolution, the direction is becoming clearer. While fully open and fully closed approaches will continue to coexist, the industry is gravitating toward standardization of key interfaces that enable different systems to work together.
This doesn't mean proprietary models or services will disappear—far from it. Just as Google built a successful business on the open web, companies will continue to develop valuable proprietary AI offerings. The difference is that these offerings will increasingly need to play well with others through standard interfaces.
As we build this future, Billmann’s insight rings true -
"we are still building all of those products and all of those experiences for the humans."
Technology serves human needs and values, and interoperability serves those needs by giving people more choice, flexibility, and control over their digital lives.
The AI agent revolution isn't about replacing human agency but enhancing it. Open, interoperable standards ensure that enhancement belongs to all of us—not just to those who control the platforms.
That's a future worth building together.